Training Data: The Overlooked Problem Of Modern AI
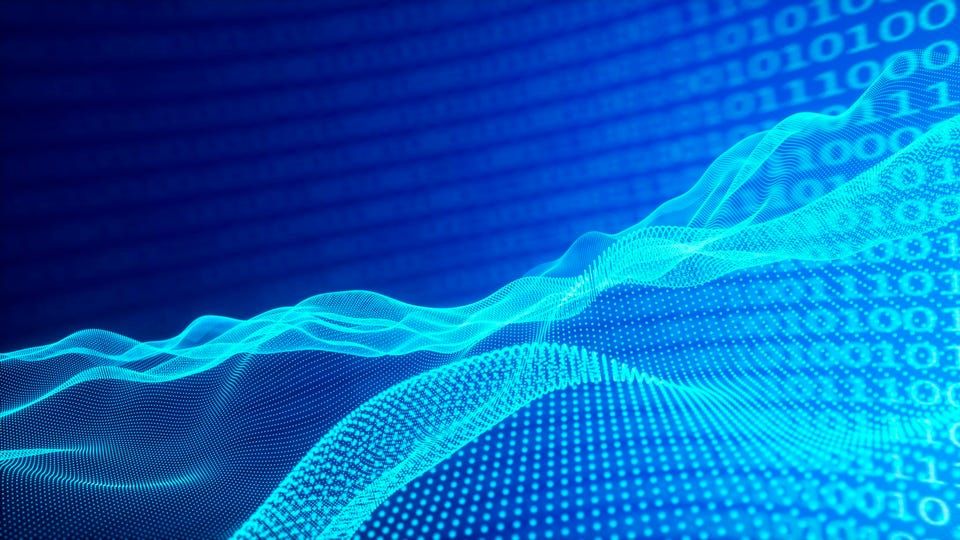
The AI market is booming, with new startups raising millions of investments in AI every day. For example, investors poured nearly $18 billion into AI in Q3 2021—three times as much as in Q1 2020. This growth is fueled by the development of cloud solutions and open-source machine learning models that have made AI technologies more accessible to many players in the market, with Brooking Institute writing that “open source software quietly affects nearly every issue in AI.”
Indeed, AI stands on three key pillars: algorithms, hardware and data. You collect large amounts of data, then using the methods of machine learning, algorithms learn to find inter-dependencies among these pieces of data and then reproduce this logic on every new piece of data they meet. This is what is now called AI (artificial intelligence).
AI From Nefertiti To Alexa
Learning from data isn’t new. Ancient Egyptians used long-term observations to predict the level of water in the Nile river. It means they were into something we would today call statistical predictive models.
The era of modern AI started with the rise of big data. Once you have large amounts of logged structured data—be it clicks on the products in an online store, time spent on a certain webpage in a browser, or percentage of paid credits in a bank—data science steps in. Building models to predict outcomes like loan return rate or success of an ad campaign becomes a standard task for a data science team.
However, in reality, the data is often either not structured or, even worse, does not exist at all. For example, a self-driving car will only be able to detect pedestrians in the street after the model has been fed with thousands of examples, including images of the streets with every pedestrian carefully highlighted and labeled.
Further, a search engine will only learn how to rank the most relevant sites on top after “seeing” millions of pairs matching user queries and web pages documents, judged by the relevance of the match.
Meanwhile, a voice assistant will only learn to correctly activate after the model analyses thousands of hours of speech recordings made by different voices and accents amidst surrounding noises.
And a brand new AI-powered app will only be able to recommend you the trendiest outfit if it is trained on a vast and up-to-date dataset of the trendiest outfits. And if the creators of the App fail to update their dataset every season, before long, it will be suggesting something that had gone out of fashion seasons ago.
All the magic and power of artificial intelligence has a natural glass ceiling—and that ceiling is data.
Is It Really Artificial?
The irony is that artificial intelligence is neither truly intelligent nor truly artificial. For one, it is heavily dependent on human efforts. In all the above-mentioned cases, the first thing you need is the effort of a human being. Interestingly, even with the rise of new self-supervised learning approaches, the need for human-powered data labeling only continues to grow: You still need data to fine-tune and validate automatically generated solutions.
It All Starts With A Dataset
With other components of AI equally available for all the players on the market, it is data that really makes your AI solution stand out from the competition. You need to be able to get unique data, label it in the most time- and cost-effective way and keep the solution regularly monitored after being deployed to production. Therefore, those who can set up regular processes of validating and updating their solutions based on real-life data get a more reliable solution.
Yet, for some reason, the importance of data labeling had been hugely underestimated and treated as a nontechnological, ineffective, and boring management task. As a result, even the most tech-heavy companies have outsourced data labeling solutions to nontech third-party vendors, according to data from our company’s survey.
Data Labeling Of The New Generation
It is only recently with the boom of AI in traditionally offline industries (such as retail or agrotech or healthcare), and the increasing need in human-powered training data on a large scale, that the industry started to seek new ways of solving the old problem. That is why in recent years, we’ve seen a series of unicorns in the data labeling domain. These solutions treat data production as part of an automated technological process with the goal of delivering training datasets for AI in the most advanced way possible.
Key Takeaways
Labeling data is an important part of the machine learning production process. It can be treated as an engineering and mathematical task that can be solved through technological means. Automation is an important aspect of data labeling, and it can be accomplished through a combination of human and machine efforts.
Link to the original version
https://www.forbes.com/sites/forbestechcouncil/2022/06/27/training-data-the-overlooked-problem-of-modern-ai/?sh=36fd7569218b